UPS! Seite nicht gefunden
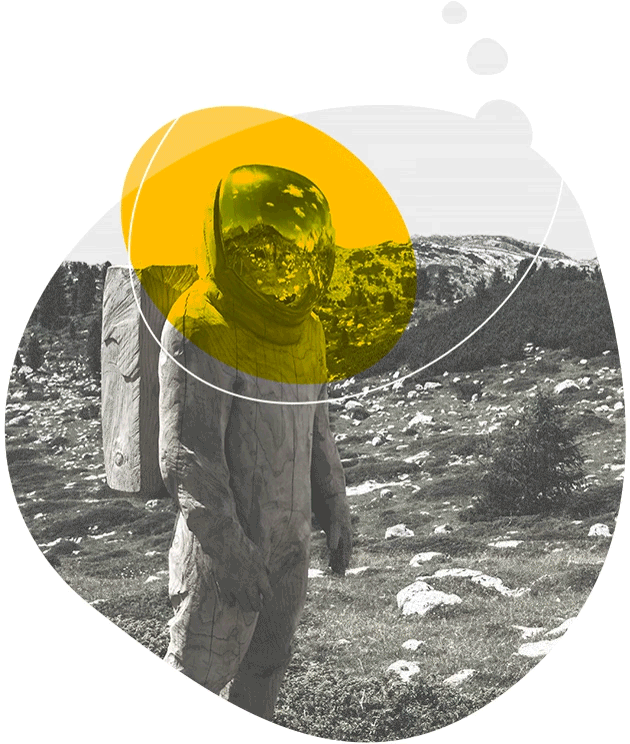
Bitte benutzen Sie die Navigation um auf die von Ihnen gewünschte Seite zu gelangen oder klicken Sie auf die Schaltfläche Zurück, um zu der vorherigen Seite zurückzukehren.
Bitte benutzen Sie die Navigation um auf die von Ihnen gewünschte Seite zu gelangen oder klicken Sie auf die Schaltfläche Zurück, um zu der vorherigen Seite zurückzukehren.